INTELLIGENT MACHINES LAB (iML)
We perform fundamental and applied research in machine learning, deep learning and related areas in computer vision and natural language processing.
More specifically, We are interested in interpretable machine learning, deep neural networks and transfer learning. The goal is to create ML models with explainability in mind, or to develop methods that can decipher existing black-box ML models. We are also interested in how ML models can learn to perform new tasks with limitted amount of labeled data; a capability that human is very good at.
Research
Keep up to date with what we're working on!
Explainable AI and NLP to help better understand issues around misinformation and vaccine hesitancy in social media
Collaboration with the Department of law and Legal Studies and the School of Journalism and Communication at Carleton U
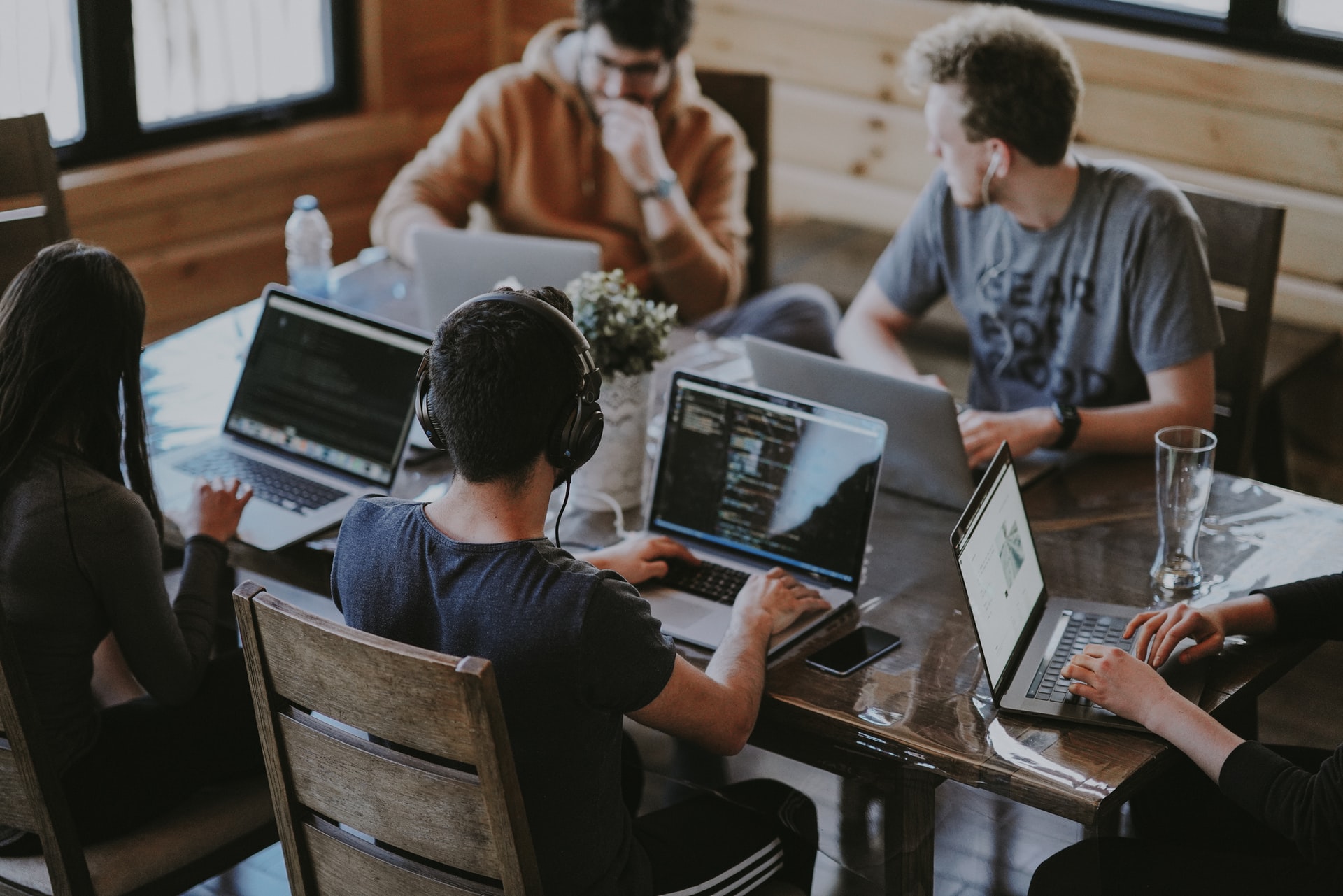
Explainable AI and NLP for assessment of functional limitations and disability services for postsecondary education
Collaboration with the Readi initiative, Accessibility Institute and The Paul Menton Centre (PMC) for Students with Disabilities.
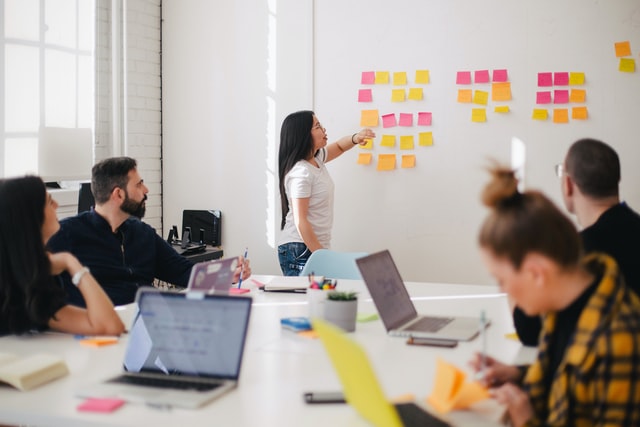
Deep learning and computer vision for communicating graphical information to visually impaired or blind individuals
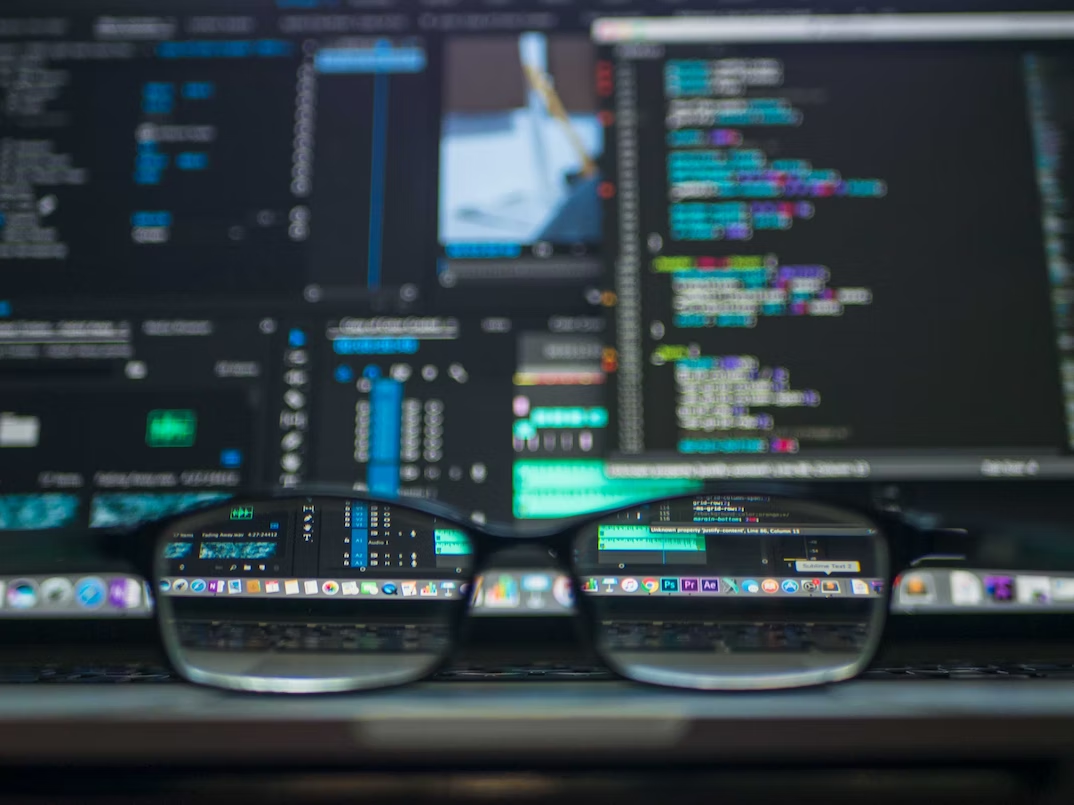
Explainable AI for predicting chronic homelessness
Collaboration with the City of Ottawa
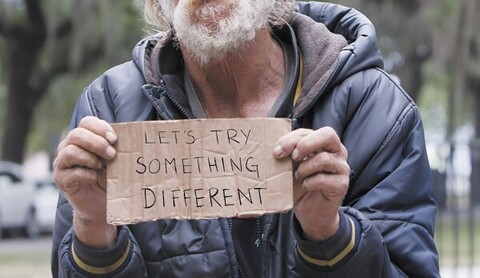
Artificial Intelligence in real-time perioperative Electrocardiogram (ECG) monitoring
Collaboration with the Ottawa hospital
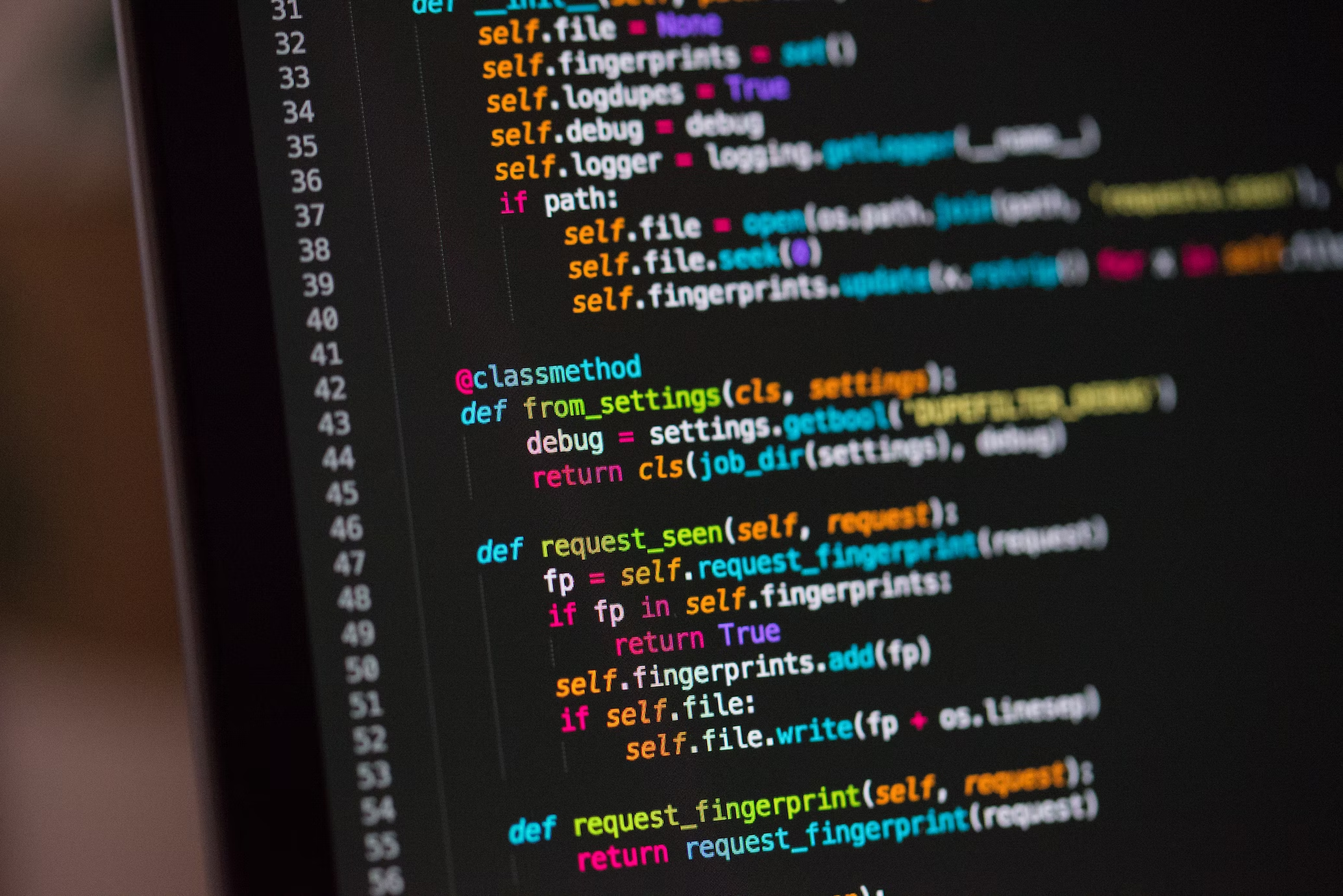
Explainable AI for predictive analytics in employee benefits insurance
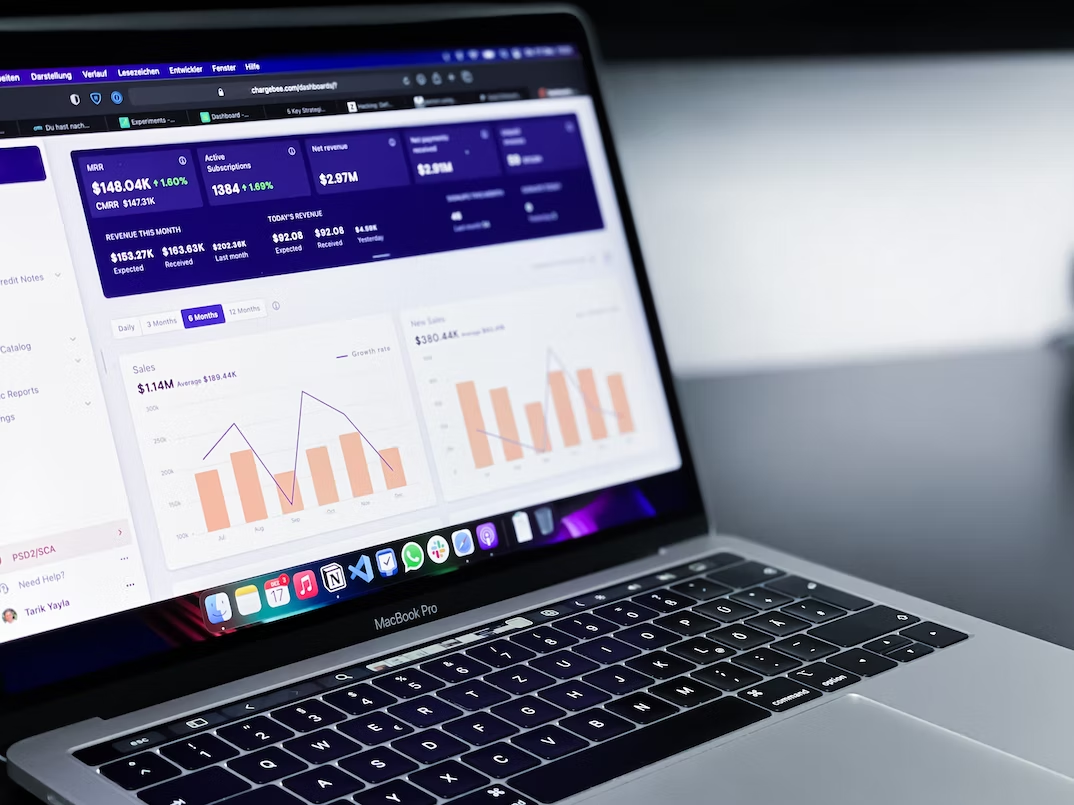
Explainable AI for analyzing EMR data
Collaboration with the Institute of Mental Health Research, Ottawa
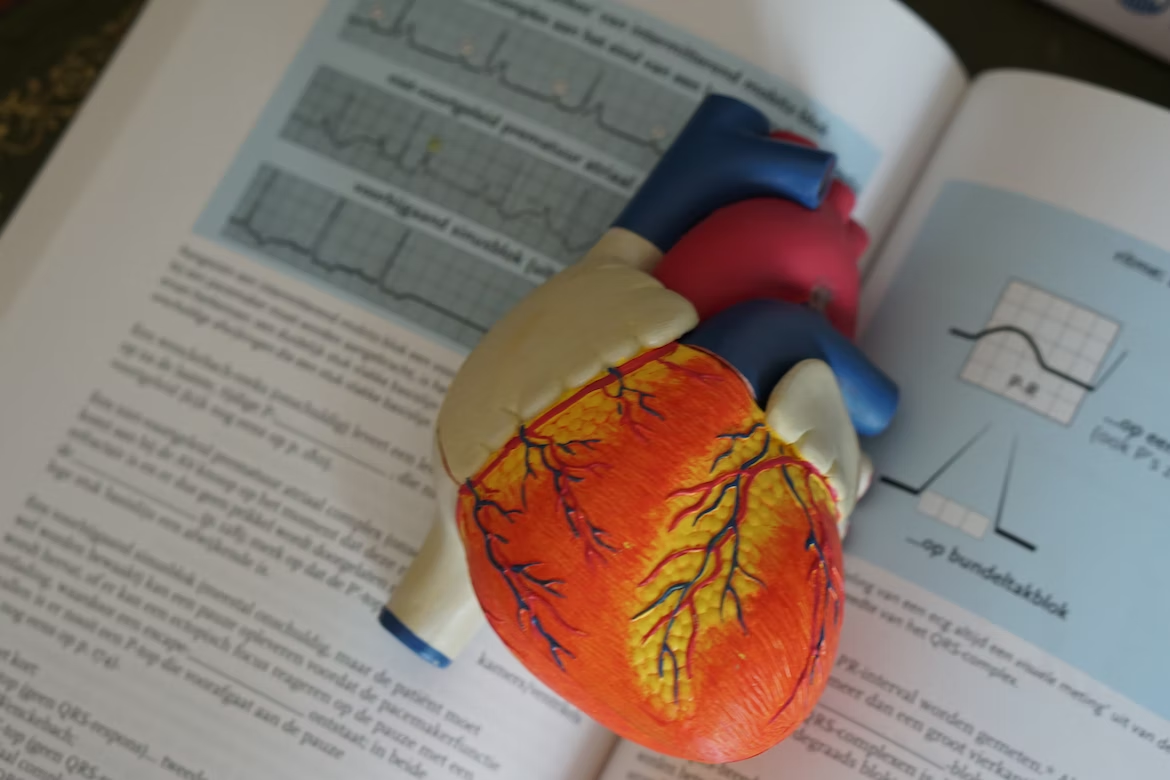
Past Projects
Biometrics, spoofing attacks and countermeasures
ML for analyzing brain signals (EEG)
ML for unobtrusive monitoring of vital physiologic parameters
ML for analyzing brain MRI images in patients with ASD
Digital tools for revitalizing endangered languages (ELK-Tech)
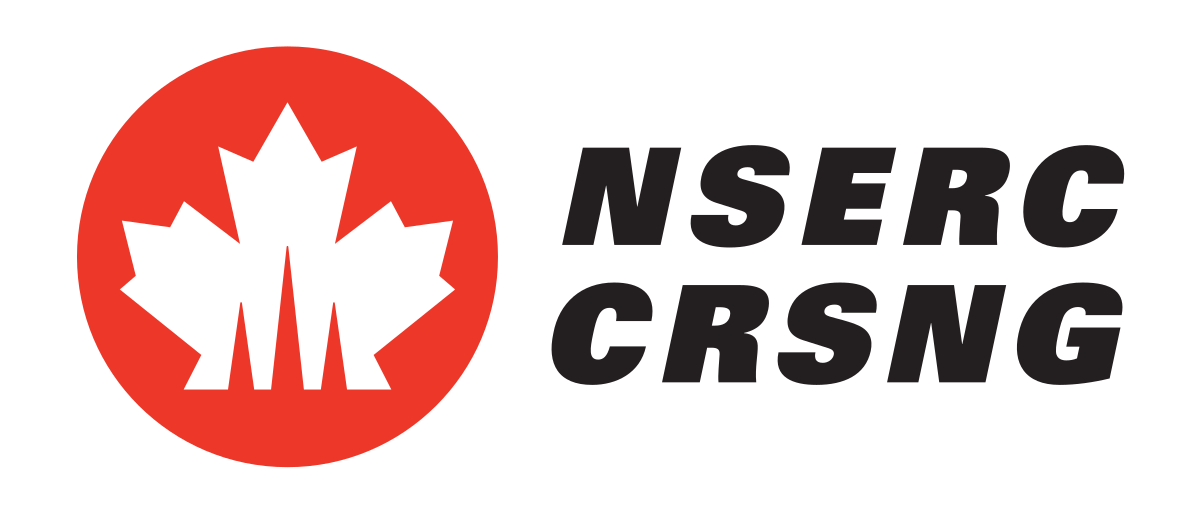
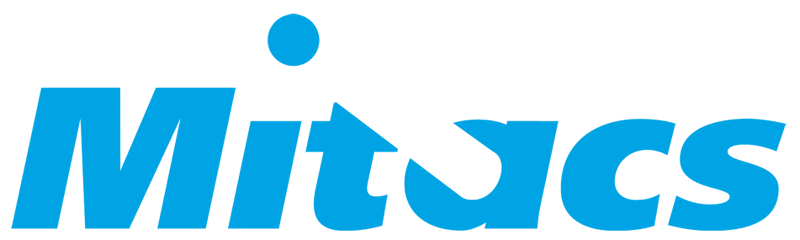
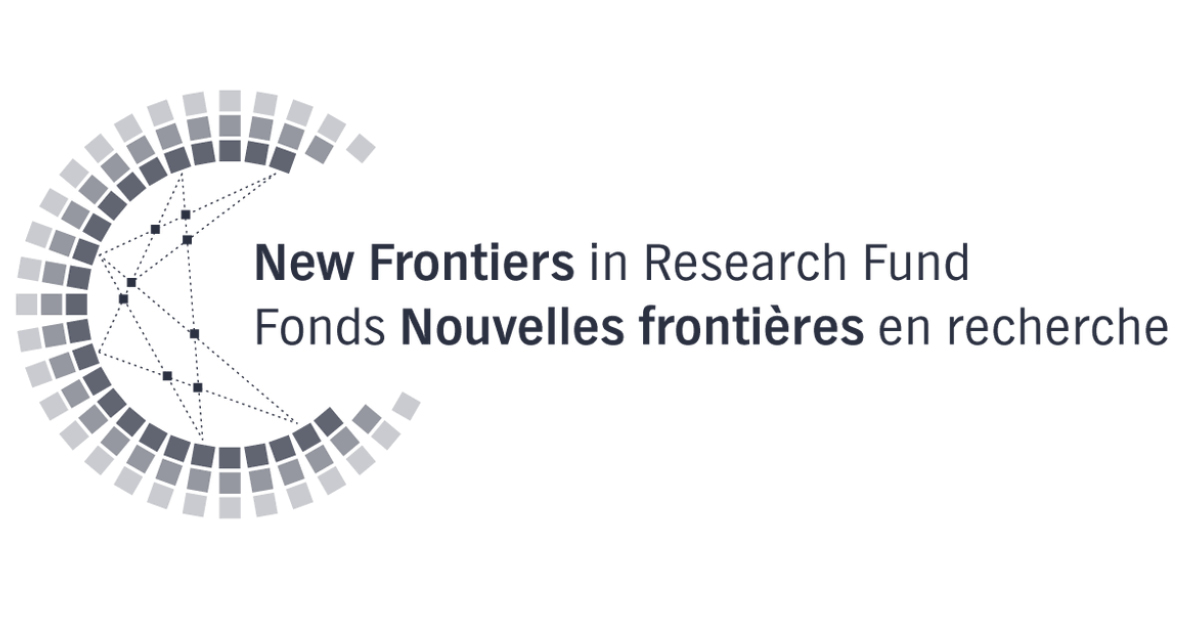
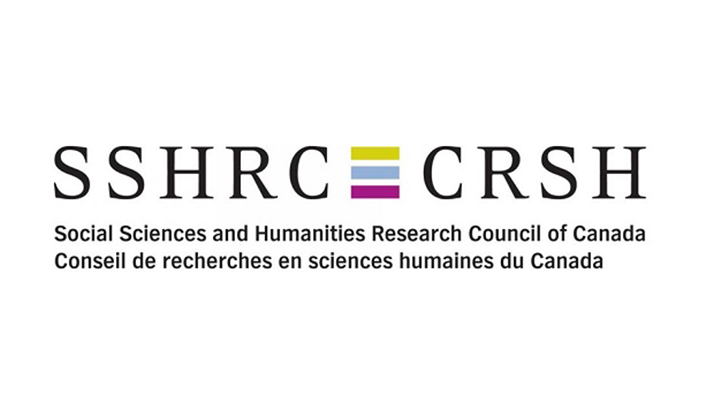
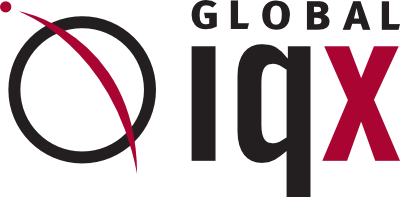
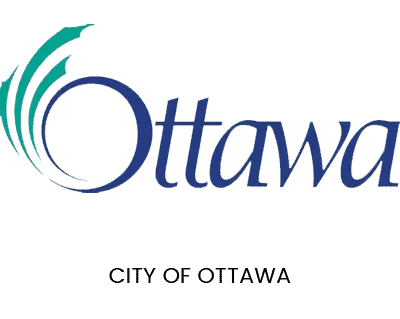
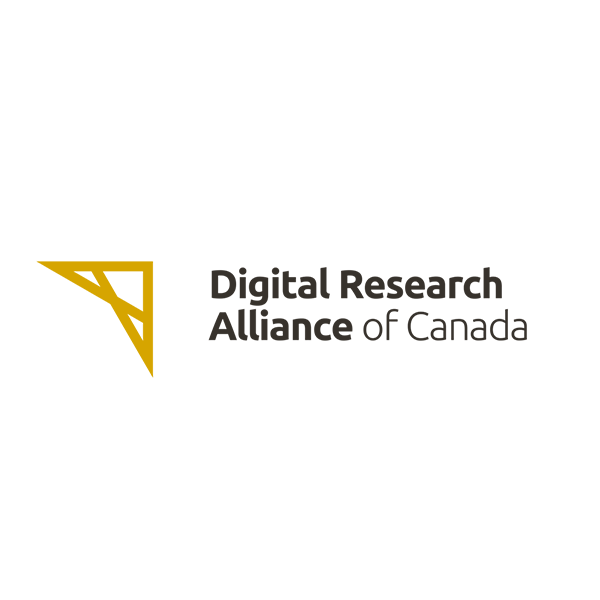
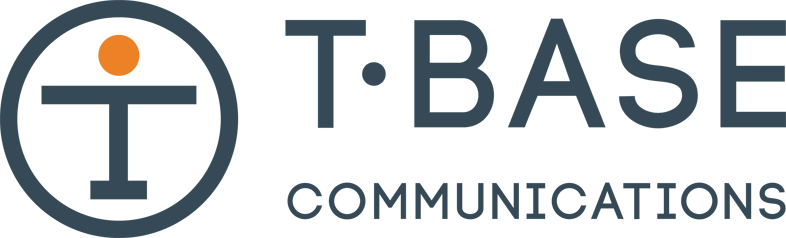
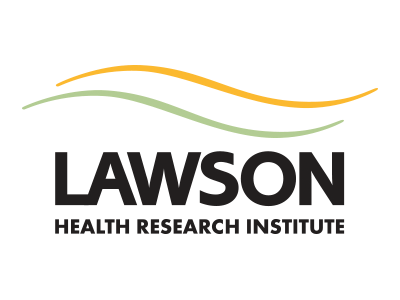
Our team
Small team. Big hearts.
Our focus is always on finding the best people to work with. Our bar is high, but you look ready to take on the challenge.
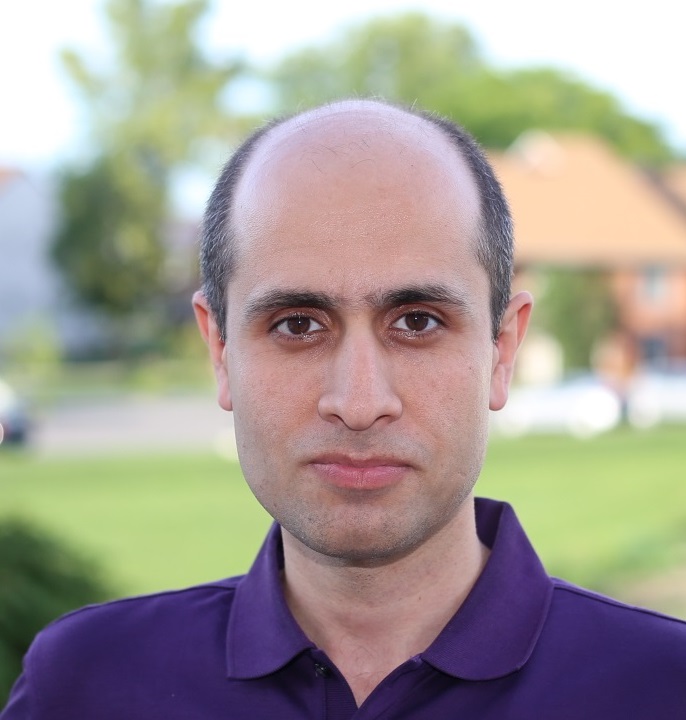
Majid Komeili
Director
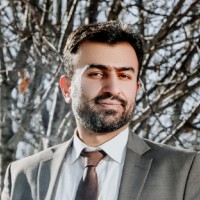
Abbas Akkasi
Postdoctoral Fellow (with Boris Vukovic and Kathleen Fraser)
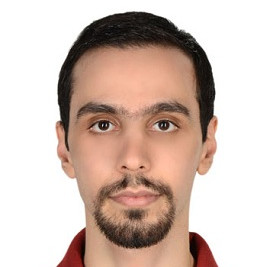
Seyed Omid Davoudi
PhD (with Frank Dehne)
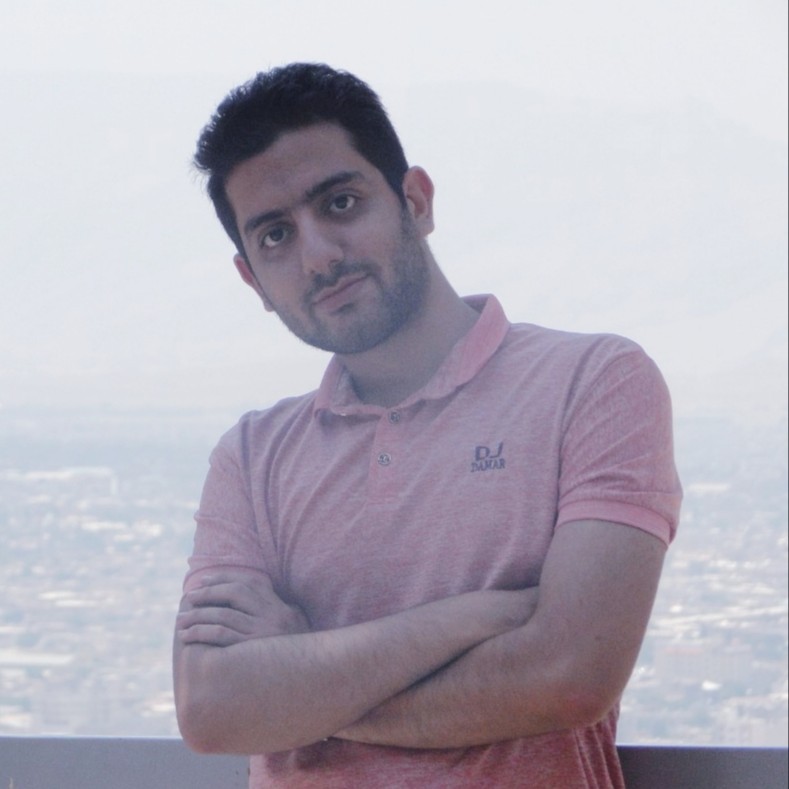
Mohammad Reza Zarei
PhD (with Frank Dehne)
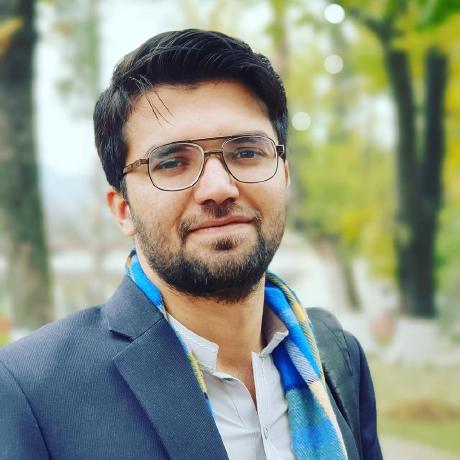
Adnan Khan
PhD (From Fall 2023)
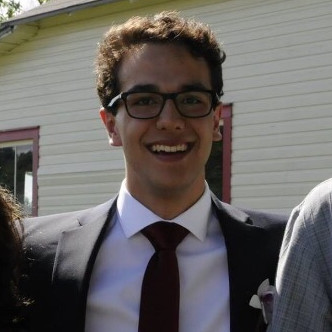
Mitchell Chatterjee
MCS (with Adrian Chan)
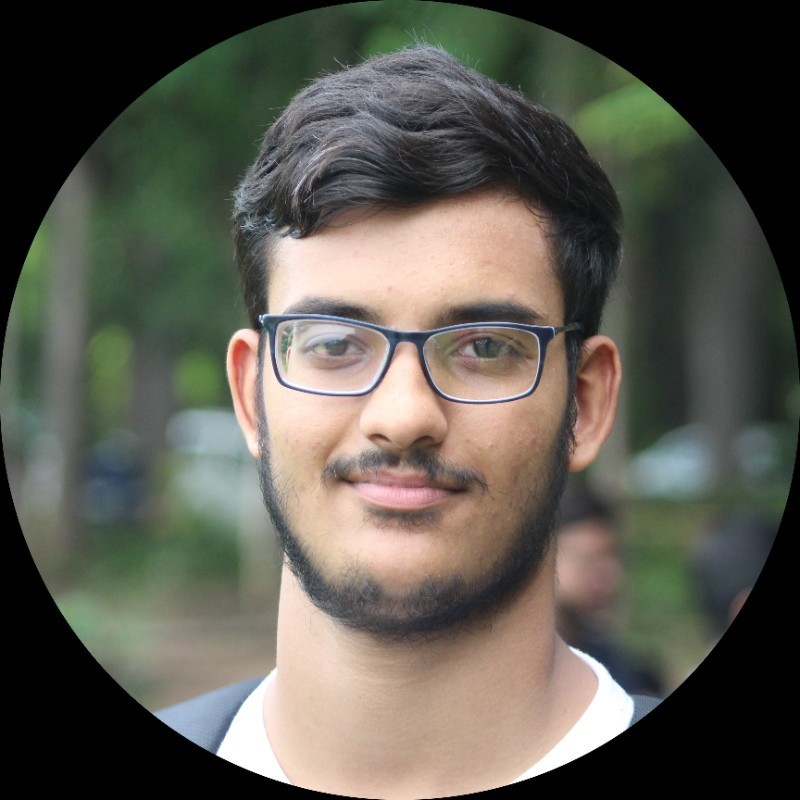
Rakshil Kevadiya
MCS (with Boris Vukovic and Kathleen Fraser)
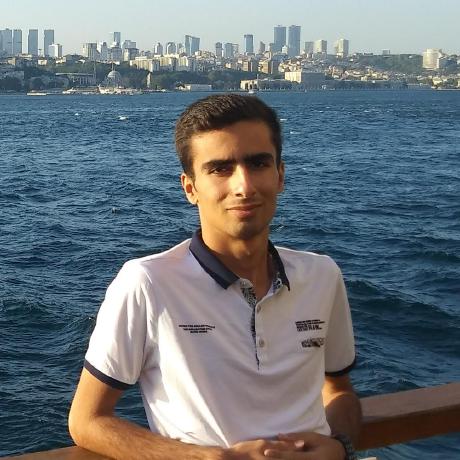
Alireza Choubineh
MCS
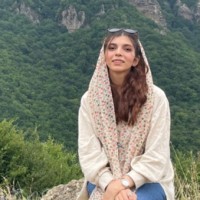
Hoda Vafaeesefat
MCS (From Fall 2023)
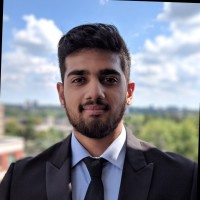
Saurabh Gummaraj Kishore
Honors Project
Past Grad Students
Aatreyi Pranavbhai Mehta, Winter 2023, MCS, moved on to Razor Sharp Consulting
Galen O'Shea, Winter 2023, MCS, moved on to Mission Control
Mohammad Mahdi Heydari Dastjerdi, Summer 2022, MCS, moved on to Paphus Solutions
Mohammad Nokhbeh Zaeem, Winter 2021, MCS, moved on to SoundHound Inc
Siraj Ahmed, Fall 2020, MCS, U Ottawa, Co-supervised with Prof. J. Park, moved on to Braiyt AI Inc
Abhijeet Chauhan, 2020, MCS, moved on to IMRSV Data Labs
Past Undergrad Students
David Hobson, Winter 2023, Honors Thesis
Kailash Balakrishnan, Winter 2023, Honors Project
Jesse Mendoza, Honors Project
Hilaire Djani, Honors Thesis
Tim Elliott, Honors Project
Juntong He, Honors Project
Qixiang Luan, Honors Project
M. Kazman, Fall 2021, Honors Project.
A. Ong, Fall 2021, Honors Project.
J. Woo, Summer 2021, Honors Project.
I. Nicolaev, Summer 2021, Honors Project.
M. Kazman, Summer 2021, Honors Project.
J. Geng, Winter 2021, Honors Project.
Y. Song, Winter 2021, Honors Project.
K. Zhen, Winter 2021, Honors Project.
H. Le, Fall 2020, Honors Project.
Y. Gao, Fall 2020, Honors Project.
T. Cao, Fall 2020, Honors Project.
Y. Chen, Fall 2020, Honors Project.
V. Nguyen, Summer 2020, Honors Project.
J. Danovitch, Winter 2020, Honors Thesis.
M. Kuzmenko, Winter 2020, Honors Project.
L. Wise, Winter 2020, Honors Project.
L. Koftinow-Mikan, Fall 2019, Honors Project.
X. Liu, Fall 2019, Honors Project.
G. O'Shea, Summer 2019, Honors Project.
L. Colwell, Summer 2019, DSRI internship.
K. Causton, Summer 2019, Honors Project (with Oliver).
Y. Yamanaka, Winter 2019, Honors Project.
S. Kudolo, Winter 2019, Honors Project.
L. Gruska, Winter 2019, Honors Project.
L. He, Winter 2019, Honors Project.
Joining/Volunteering
APPLYING FOR MSC OR PHD:
MSc and PhD applicants who are interested in my research are encouraged to contact me via email.
Prerequisites: A good candidate should have background in probability and linear algebra, and have had courses in Machine Learning or related areas including Computer Vision and Natural Language Processing.
Prospective MSc and PhD students who are applying to the School of Computer Science at Carleton University and are interested in my research are encouraged to indicate my name as their preferred research supervisor.
Please note that due to the volume of emails I receive, I am not able to respond to all.
Undergrad students at Carleton University who are interested in doing their Honours project/thesis with me, are encouraged to contact me via email.
Publications
Galen O'Shea, Majid Komeili (2023) , “SuperVision: Self-Supervised Super-Resolution for Appearance-Based Gaze Estimation”, NeurIPS 2023 Gaze Meets ML Workshop, PDF
Gaze estimation is a valuable tool with a broad range of applications in various fields, including medicine, psychology, virtual reality, marketing, and safety. Therefore, it is essential to have gaze estimation software that is cost-efficient and high-performing. Accurately predicting gaze remains a difficult task, particularly in real-world situations where images are affected by motion blur, video compression, and noise. Super-resolution (SR) has been shown to remove these degradations and improve image quality from a visual perspective. This work examines the usefulness of super-resolution for improving appearance-based gaze estimation and demonstrates that not all SR models preserve the gaze direction. We propose a two-step framework for gaze estimation based on the SwinIR super-resolution model. The proposed method consistently outperforms the state-of-the-art, particularly in scenarios involving low-resolution or degraded images. Furthermore, we examine the use of super-resolution through the lens of self-supervised learning for gaze estimation and propose a novel architecture “SuperVision” by fusing an SR backbone network to a ResNet18. While only using 20% of the data, the proposed SuperVision architecture outperforms the state-of-the-art GazeTR method by 15.5%.
Mai Shaaban, Abbas Akkasi, Adnan Khan, Majid Komeili, Mohammad Yaqub (2023) , “Fine-Tuned Large Language Models for Symptom Recognition from Spanish Clinical Text”, BioCreative, PDF
The accurate recognition of symptoms in clinical reports is significantly important in the fields of healthcare and biomedical natural language processing. These entities serve as essential building blocks for clinical information extraction, enabling retrieval of critical medical insights from vast amounts of textual data. Furthermore, the ability to identify and categorize these entities is fundamental for developing advanced clinical decision support systems, aiding healthcare professionals in diagnosis and treatment planning. In this study, we participated in SympTEMIST – a shared task on detection of symptoms, signs and findings in Spanish medical documents. We combine a set of large language models finetuned with the data released by the task's organizers.
Omid Davoodi, Shayan Mohammadizadehsamakosh, Majid Komeili (2023) , “On the Interpretability of Part-Prototype Based Classifiers: A Human Centric Analysis”, Preprint
Part-prototype networks have recently become methods of interest as an interpretable alternative to many of the current black-box image classifiers. However, the interpretability of these methods from the perspective of human users has not been sufficiently explored. In this work, we have devised a framework for evaluating the interpretability of part-prototype-based models from a human perspective. The proposed framework consists of three actionable metrics and experiments. To demonstrate the usefulness of our framework, we performed an extensive set of experiments using Amazon Mechanical Turk. They not only show the capability of our framework in assessing the interpretability of various part-prototype-based models, but they also are, to the best of our knowledge, the most comprehensive work on evaluating such methods in a unified framework.
Abbas Akkasi, Kathleen C. Fraser, and Majid Komeili (2023) , “Reference-free Summarization Evaluation with Large Language Models”, IJCNLP-AACL Workshop on Evaluation and Comparison for NLP systems,
M. R. Zarei, M. Christensen, S. Everts, M. Komeili (2023) , “Vax-Culture: A Dataset for Studying Vaccine Discourse on Twitter”, International Joint Conference on Neural Networks, Code, PDF
Vaccine hesitancy continues to be a main challenge for public health officials during the COVID-19 pandemic. As this hesitancy undermines vaccine campaigns, many researchers have sought to identify its root causes, finding that the increasing volume of anti-vaccine misinformation on social media platforms is a key element of this problem. We explored Twitter as a source of misleading content with the goal of extracting overlapping cultural and political beliefs that motivate the spread of vaccine misinformation. To do this, we have collected a data set of vaccine-related Tweets and annotated them with the help of a team of annotators with a background in communications and journalism. Ultimately we hope this can lead to effective and targeted public health communication strategies for reaching individuals with anti-vaccine beliefs. Moreover, this information helps with developing Machine Learning models to automatically detect vaccine misinformation posts and combat their negative impacts. In this paper, we present Vax-Culture, a novel Twitter COVID-19 dataset consisting of 6373 vaccine-related tweets accompanied by an extensive set of human-provided annotations including vaccine-hesitancy stance, indication of any misinformation in tweets, the entities criticized and supported in each tweet and the communicated message of each tweet. Moreover, we define five baseline tasks including four classification and one sequence generation tasks, and report the results of a set of recent transformer-based models for them. The dataset and code are publicly available at https://github.com/mrzarei5/Vax-Culture.
Galen O'Shea, Majid Komeili, (2023) , “Toward Super-Resolution for Appearance-Based Gaze Estimation”, Preprint
Gaze tracking is a valuable tool with a broad range of applications in various fields, including medicine, psychology, virtual reality, marketing, and safety. Therefore, it is essential to have gaze tracking software that is cost-efficient and high-performing. Accurately predicting gaze remains a difficult task, particularly in real-world situations where images are affected by motion blur, video compression, and noise. Super-resolution has been shown to improve image quality from a visual perspective. This work examines the usefulness of super-resolution for improving appearancebased gaze tracking. We show that not all SR models preserve the gaze direction. We propose a two-step framework based on SwinIR super-resolution model. The proposed method consistently outperforms the state-of-the-art, particularly in scenarios involving low-resolution or degraded images. Furthermore, we examine the use of superresolution through the lens of self-supervised learning for gaze prediction. Self-supervised learning aims to learn from unlabelled data to reduce the amount of required labeled data for downstream tasks. We propose a novel architecture called “SuperVision” by fusing an SR backbone network to a ResNet18 (with some skip connections). The proposed SuperVision method uses 5x less labeled data and yet outperforms, by 15%, the state-of-the-art method of GazeTR which uses 100% of training data. We will make our code publicly available upon publication.
M.R. Zarei, M. Komeili, (2022) , “Interpretable Few-shot Learning with Online Attribute Selection”, Preprint
Few-shot learning (FSL) is a challenging learning problem in which only a few samples are available for each class. Decision interpretation is more important in few-shot classification since there is a greater chance of error than in traditional classification. However, most of the previous FSL methods are black-box models. In this paper, we propose an inherently interpretable model for FSL based on human-friendly attributes. Moreover, we propose an online attribute selection mechanism that can effectively filter out irrelevant attributes in each episode. The attribute selection mechanism improves the accuracy and helps with interpretability by reducing the number of participated attributes in each episode. We demonstrate that the proposed method achieves results on par with black-box few-shot-learning models on four widely used datasets. To further close the performance gap with the black-box models, we propose a mechanism that trades interpretability for accuracy. It automatically detects the episodes where the provided humanfriendly attributes are not adequate, and compensates by engaging learned unknown attributes.
M.R. Zarei, M. Komeili, (2022) , “Interpretable Concept-based Prototypical Networks for Few-Shot Learning”, ICIP, PDF
Few-shot learning aims at recognizing new instances from classes with limited samples. This challenging task is usually alleviated by performing meta-learning on similar tasks. However, the resulting models are black-boxes. There has been growing concerns about deploying black-box machine learning models and FSL is not an exception in this regard. In this paper, we propose a method for FSL based on a set of human-interpretable concepts. It constructs a set of metric spaces associated with the concepts and classifies samples of novel classes by aggregating concept-specific decisions. The proposed method does not require concept annotations for query samples. This interpretable method achieved results on a par with six previously state-of-the-art black-box FSL methods on the CUB fine-grained bird classification dataset.
O. Davoudi, M. Komeili, (2022) , “Toward Faithful Case-based Reasoning through Learning Prototypes in a Nearest Neighbor-friendly Space”, ICLR, PDF
Recent advances in machine learning have brought opportunities for the ever-increasing use of AI in the real world. This has created concerns about the black-box nature of many of the most recent machine learning approaches. In this work, we propose an interpretable neural network that leverages metric and prototype learning for classification tasks. It encodes its own explanations and provides an improved case-based reasoning through learning prototypes in an embedding space learned by a probabilistic nearest neighbor rule. Through experiments, we demonstrated the effectiveness of the proposed method in both performance and the accuracy of the explanations provided.
Siraj Ahmed, Majid Komeili, Jeongwon Park, (2022) , “Predictive Modelling of Parkinson’s Disease Progression Based on RNA-Sequence with Densely Connected Deep Recurrent Neural Networks”, nature Scientific Reports, PDF
The advent of recent high throughput sequencing technologies resulted in unexplored big data of genomics and transcriptomics that might help to answer various research questions in Parkinson’s disease (PD) progression. While the literature has revealed various predictive models that use longitudinal clinical data for disease progression, there is no predictive model based on RNA-Sequence data of PD patients. This study investigates how to predict the PD Progression for a patient’s next medical visit by capturing longitudinal temporal patterns in the RNA-Seq data. Data provided by Parkinson Progression Marker Initiative (PPMI) includes 423 PD patients without revealing any race, sex, or age information with a variable number of visits and 34,682 predictor variables for 4 years. We propose a predictive model based on deep Recurrent Neural Network (RNN) with the addition of dense connections and batch normalization into RNN layers. The results show that the proposed architecture can predict PD progression from high dimensional RNA-seq data with a Root Mean Square Error (RMSE) of 6.0 and a rank-order correlation of (r = 0.83, p < 0.0001) between the predicted and actual disease status of PD.
M. Nokhbeh Zaeem, M. Komeili, (2022) , “Cause and Effect: Hierarchical Concept-based Explanation of Neural Networks”, IEEE Transactions on Neural Networks and Learning Systems, Preprint
In many scenarios, human decisions are explained based on some high-level concepts. In this work, we take a step in the interpretability of neural networks by examining their internal representation or neuron’s activations against concepts. A concept is characterized by a set of samples that have specific features in common. We propose a framework to check the existence of a causal relationship between a concept (or its negation) and task classes. While the previous methods focus on the importance of a concept to a task class, we go further and introduce four measures to quantitativTITLEely determine the order of causality. Moreover, we propose a method for constructing a hierarchy of concepts in the form of a conceptbased decision tree which can shed light on how various concepts interact inside a neural network towards predicting output classes. Through experiments, we demonstrate the effectiveness of the proposed method in explaining the causal relationship between a concept and the predictive behaviour of a neural network as well as determining the interactions between different concepts through constructing a concept hierarchy.
O. Davoudi, M. Komeili, (2021) , “Feature-Based Interpretable Reinforcement Learning based on State-Transition Models”, IEEE International Conference on Systems, Man, and Cybernetics, PDF
Growing concerns regarding the operational usage of AI models in the real-world has caused a surge of interest in explaining AI models’ decisions to humans. Reinforcement Learning is not an exception in this regard. In this work, we propose a method for offering local explanations on risk in reinforcement learning. Our method only requires a log of previous interactions between the agent and the environment to create a state-transition model. It is designed to work on RL environments with either continuous or discrete state and action spaces. After creating the model, actions of any agent can be explained in terms of the features most influential in increasing or decreasing risk or any other desirable objective function in the locality of the agent. Through experiments, we demonstrate the effectiveness of the proposed method in providing such explanations
K. C. Fraser, M. Komeili, (2021) , “Measuring Cognitive Status from Speech in a Smart Home Environment”, IEEE Instrumentation and Measurement Magazine, PDF
The population is aging, and becoming more tech-savvy. The United Nations predicts that by 2050, one in six people in the world will be over age 65 (up from one in 11 in 2019), and this increases to one in four in Europe and Northern America. Meanwhile, the proportion of American adults over 65 who own a smartphone has risen 24 percentage points from 2013-2017, and the majority have Internet in their homes. Smart devices and smart home technology have profound potential to transform how people age, their ability to live independently in later years, and their interactions with their circle of care. Cognitive health is a key component to independence and well-being in old age, and smart homes present many opportunities to measure cognitive status in a continuous, unobtrusive manner. In this article, we focus on speech as a measurement instrument for cognitive health. Existing methods of cognitive assessment suffer from a number of limitations that could be addressed through smart home speech sensing technologies. We begin with a brief tutorial on measuring cognitive status from speech, including some pointers to useful open-source software toolboxes for the interested reader. We then present an overview of the preliminary results from pilot studies on active and passive smart home speech sensing for the measurement of cognitive health, and conclude with some recommendations and challenge statements for the next wave of work in this area, to help overcome both technical and ethical barriers to success.
M. Nokhbeh Zaeem, M. Komeili, (2021) , “Cause and Effect: Concept-based Explanation of Neural Networks”, IEEE International Conference on Systems, Man, and Cybernetics, Code, PDF
In many scenarios, human decisions are explained based on some high-level concepts. In this work, we take a step in the interpretability of neural networks by examining their internal representation or neuron’s activations against concepts. A concept is characterized by a set of samples that have specific features in common. We propose a framework to check the existence of a causal relationship between a concept (or its negation) and task classes. While the previous methods focus on the importance of a concept to a task class, we go further and introduce four measures to quantitatively determine the order of causality. Through experiments, we demonstrate the effectiveness of the proposed method in explaining the relationship between a concept and the predictive behaviour of a neural network.
A. Chauhan, O. Davoudi M. Komeili, (2021) , “Multi-scale Deep Nearest Neighbors”, International Joint Conference on Neural Networks, PDF
We propose a differentiable loss function for learning an embedding space by minimizing the upper bound of the leave-one-out classification error rate of 1-nearest neighbor classification error in the latent space. To evaluate the resulting space, in addition to the classification performance, we examine the problem of finding subclasses. In many applications, it is desired to detect unknown subclasses that might exist within known classes. For example, discovering subtypes of a known disease may help develop customized treatments. Analogous to the hierarchical clustering, subclasses might exist on different scales. The proposed method provides a mechanism to target subclasses in different scales.
M. Komeili, N. Armanfard, D. Hatzinakos, (2020) , “Multiview Feature Selection for Single-view Classification”, IEEE Transactions on Pattern Analysis and Machine Intelligence, Code, PDF
—In many real-world scenarios, data from multiple modalities (sources) are collected during a development phase. Such data are referred to as multiview data. While additional information from multiple views often improves the performance, collecting data from such additional views during the testing phase may not be desired due to the high costs associated with measuring such views or, unavailability of such additional views. Therefore, in many applications, despite having a multiview training data set, it is desired to do performance testing using data from only one view. In this paper, we present a multiview feature selection method that leverages the knowledge of all views and use it to guide the feature selection process in an individual view. We realize this via a multiview feature weighting scheme such that the local margins of samples in each view are maximized and similarities of samples to some reference points in different views are preserved. Also, the proposed formulation can be used for cross-view matching when the view-specific feature weights are pre-computed on an auxiliary data set. Promising results have been achieved on nine real-world data sets as well as three biometric recognition applications. On average, the proposed feature selection method has improved the classification error rate by 31% of the error rate of the state-of-the-art.
M. Komeili, C. Pou-Prom, D. Liaqat, K. C. Fraser, M. Yancheva, F. Rudzicz, (2019) , “Talk2Me: Automated Linguistic Data Collection for Personal Assessment”, PLoS One, Code, PDF
Language is one the earliest capacities affected by cognitive change. To monitor that change longitudinally, we have developed a web portal for remote linguistic data acquisition, called Talk2Me, consisting of a variety of tasks. In order to facilitate research in different aspects of language, we provide baselines including the relations between different scoring functions within and across tasks. These data can be used to augment studies that require a normative model; for example, we provide baseline classification results in identifying dementia. These data are released publicly along with a comprehensive open-source package for extracting approximately two thousand lexico-syntactic, acoustic, and semantic features. This package can be applied arbitrarily to studies that include linguistic data. To our knowledge, this is the most comprehensive publicly available software for extracting linguistic features. The software includes scoring functions for different tasks.
N Armanfard, M. Komeili, JP Reilly, J Connoly, (2019) , “A Machine Learning Framework for Automatic and Continuous MMN Detection with Preliminary Results for Coma Outcome Prediction”, IEEE Journal of Biomedical and Health Informatics, vol. 23, issue 4, pp. 1794 -1804,
S. J. Haghighi, M. Komeili, D. Hatzinakos, H. El-Beheiry, (2018) , “40-Hz ASSR for Measuring Depth of Anaesthesia During Induction Phase”, IEEE Journal of Biomedical and Health Informatics, vol. 22, issue 6, pp. 1871 - 1882,
M. Komeili, N. Armanfard, D. Hatzinakos, (2018) , “Liveness Detection and Automatic Template Updating using Fusion of ECG and Fingerprint”, IEEE Transactions on Information Forensics & Security, vol. 13, issue 7, pp. 1810 - 1822, PDF
Fingerprint has been extensively used for biometric recognition around the world. However, fingerprints are not secrets and an adversary can synthesis a fake finger to spoof the biometric system. The mainstream of the current fingerprint spoof detection methods are basically binary classifier trained on some real and fake samples. While they perform well on detecting fake samples created by using the same methods used for training, their performance degrades when encountering fake samples created by a novel spoofing method. In this paper, we approach the problem from a different perspective by incorporating ECG. Compare with the conventional biometrics, stealing someone’s ECG is far more difficult if not impossible. Considering that ECG is a vital signal and motivated by its inherent liveness, we propose to combine it with a fingerprint liveness detection algorithm. The combination is natural as both ECG and fingerprint can be captured from fingertips. In the proposed framework, ECG and fingerprint are combined not only for authentication purpose but also for liveness detection. We also examine automatic template updating using ECG and fingerprint. In addition, we propose a stopping criterion that reduces the average waiting time for signal acquisition. We have performed extensive experiments on LivDet2015 database which is presently the latest available liveness detection database and compare the proposed method with six liveness detection methods as well as twelve participants of LivDet2015 competition. The proposed system has achieved a liveness detection EER of 4.2% incorporating only 5 seconds of ECG. By extending the recording time to 30 seconds, liveness detection EER reduces to 2.6% which is about 4 times better than the best of six comparison methods. This is also about 2 times better than the best results achieved by participants of LivDet2015 competition.
N. Armanfard, J. P. Reilly, M. Komeili, (2018) , “Logistic Localized Modeling of the Sample Space for Feature Selection and Classification”, IEEE Transactions on Neural Networks and Learning Systems, vol. 29, issue 5, pp. 1396 - 1413,
N. Armanfard, M. Komeili, A. Mihailidis (2018) , “Development of a Smart Home Package for Unobtrusive physiological Monitoring”, IEEE. 40th International Engineering in Medicine and Biology Conference, United States,
A. Kushki, M. Komeili, S. Panahandeh, E. Anagnostou, J. Lerch. (2018) , “Examining Associations Between Brain Morphology and Social Function in ASD, ADHD, OCD, and typical development using Machine Learning: Analysis of POND Network Data”, INSAR 2018, International Society for Autism Research, Netherlands,
M. Komeili, W. Louis, N. Armanfard, D. Hatzinakos, (2017) , “Feature Selection for Non-stationary Data: Application to Human Recognition using Medical Biometrics”, IEEE Transactions on Cybernetics, vol. 48, issue 5, pp. 1446 - 1459, PDF
ECG and TEOAE are among the physiological signals that have attracted significant interest in biometric community due to their inherent robustness to replay and falsification attacks. However, they are time-dependent signals and this makes them hard to deal with in across-session human recognition scenario where only one session is available for enrollment. This paper presents a novel feature selection method to address this issue. It is based on an auxiliary dataset with multiple sessions where it selects a subset of features that are more persistent across different sessions. It uses local information in terms of sample margins while enforcing an across-session measure. This makes it a perfect fit for aforementioned biometric recognition problem. Comprehensive experiments on ECG and TEOAE variability due to time lapse and body posture are done. Performance of the proposed method is compared against seven state-of-the-art feature selection algorithms as well as another six approaches in the area of ECG and TEOAE biometric recognition. Experimental results demonstrate that the proposed method performs noticeably better than other algorithms.
N. Armanfard, J. P. Reilly, M. Komeili, (2016) , “Local Feature Selection for Data Classification”, IEEE Transactions on Pattern Analysis and Machine Intelligence, vol. 38, no. 6, pp. 1217-1227,
W. Louis, M. Komeili, D. Hatzinakos, (2016) , “Continuous Authentication using One Dimensional Multi-Resolution Local Binary Patterns”, IEEE Transactions on Information Forensics & Security, vol. 11, no. 12, pp 2818-2832, PDF
The objective of a continuous authentication system is to continuously monitor the identity of subjects using biometric systems. In this paper, we proposed a novel feature extraction and a unique continuous authentication strategy and technique. We proposed One-Dimensional Multi-Resolution Local Binary Patterns (1DMRLBP), an online feature extraction for one-dimensional signals. We also proposed a continuous authentication system, which uses sequential sampling and 1DMRLBP feature extraction. This system adaptively updates decision thresholds and sample size during run-time. Unlike most other local binary patterns variants, 1DMRLBP accounts for observations’ temporal changes and has a mechanism to extract one feature vector that represents multiple observations. 1DMRLBP also accounts for quantization error, tolerates noise, and extracts local and global signal morphology. This paper examined electrocardiogram signals. When 1DMRLBP was applied on the University of Toronto database (UofTDB) 1,012 single session subjects database, an equal error rate (EER) of 7.89% was achieved in comparison to 12.30% from a state-of-the-art work. Also, an EER of 10.10% was resulted when 1DMRLBP was applied to UofTDB 82 multiple sessions database. Experiments showed that using 1DMRLBP improved EER by 15% when compared with a biometric system based on raw time-samples. Finally, when 1DMRLBP was implemented with sequential sampling to achieve a continuous authentication system, 0.39% false rejection rate and 1.57% false acceptance rate were achieved.
S. J. Haghighi, M. Komeili, D. Hatzinakos, (2016) , “Predicting the Depth of Anaesthesia with 40-Hz ASSR”, IEEE 29th Canadian Conference on Electrical and Computer Engineering (CCECE), Vancouver, Canada,
N. Armanfard, M. Komeili, J. P. Reilly, John F. Connolly, (2016) , “Automatic and continuous assessment of ERPs for Mismatch Negativity detection”, 38th Annual International Conference of the IEEE Engineering in Medicine and Biology Society (EMBC), , Orlando, FL, USA,
M. Komeili, W. Louis, N. Armanfard, D. Hatzinakos, (2016) , “Human Recognition using Electrocardiogram Signals: From Rest to Exercise”, IEEE 29th Canadian Conference on Electrical and Computer Engineering (CCECE), Vancouver, Canada,
N. Armanfard, M. Komeili, J. P. Reilly, L. Pino, (2016) , “Vigilance lapse identification using sparse EEG electrode arrays”, IEEE 29th Canadian Conference on Electrical and Computer Engineering (CCECE), Vancouver, Canada,
W. Louis, M. Komeili, D. Hatzinakos, (2016) , “Real-time Heartbeat Outlier Removal in Electrocardiogram (ECG) Biometric System”, Electrical and Computer Engineering (CCECE), IEEE 29th Canadian Conference on, Vancouver, Canada,
M. Komeili, N. Armanfard, D. Hatzinakos, A. N. Venetsanopoulos, (2015) , “Feature Selection from Multisession Electrocardiogram Signals for Identity Verification”, Electrical and Computer Engineering (CCECE), 2015 IEEE 28th Canadian Conference on, Halifax, Canada,
N. Armanfard, M. Komeili, E. Kabir, (2012) , “TED: A Texture-Edge Descriptor for Pedestrian Detection in Video Sequences”, Pattern Recognition, vol. 45, no.3, pp. 983-992,
Patents
M. Komeili, N. Armanfard, D. Hatzinakos , “An Expert System for Fingerprint Spoof Detection”, International application number CA2019050141, Patent Cooperation Treaty (PCT), Feb. 2019,
N. Armanfard, M. Komeili, J. P. Reilly, John F. Connolly , “Expert System for Automatic, Continuous Coma Patient Assessment and Outcome Prediction”, U.S. Provisional Patent, USPTO serial no. 62/509,986, May 2017,
Contact details
613-520-2600 ext. 6098
majidkomeili@cunet.carleton.ca
5422 Herzberg Laboratories, Carleton University 1125 Colonel By Drive, Ottawa Ontario, Canada, K1S 5B6